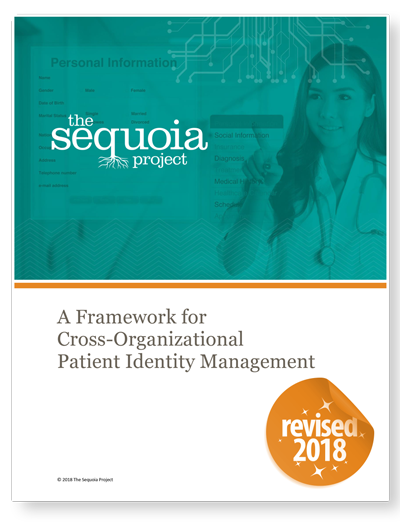
The healthcare industry is making significant progress on technical interoperability, but we continue to fall short of the promise of true health information exchange. Until we can consistently send and receive accurate and useful patient data nationwide, we will fail to realize the documented benefits of well-executed health information exchanges: improvements in clinical decision making and patient safety, business process improvement, and support for value-based payment. Among the remaining challenges to successful nationwide exchange is patient matching across organizational boundaries.
The inability to consistently and accurately match patient data creates a number of problems for physicians and other healthcare providers. Providers may have an incomplete view of a patient’s medical history, care may not be well coordinated, patient records may be overlaid, unnecessary testing or improper treatment may be ordered, and patient confidence may be damaged or diminished.
In addition, providers may experience a number of clinical workflow inefficiencies that are costly. Those include prolonged troubleshooting to find the correct patient record, a reversion to manual telephone and fax information exchange workflows, waiting for a duplicate lab test order, or a manual effort to fix a patient record match.
To address these critical patient matching and identity management issues, The Sequoia Project, in collaboration with the Care Connectivity Consortium (CCC), has developed a framework for future growth and improvement that will shed light on several key topics:
Following publication of the proposed framework, The Sequoia Project convened a Patient Identity Management Workgroup, inviting members and non-members. Comprising of industry and government experts, the workgroup was charged with dispositioning public comments to develop final recommendations for improved patient identity management in a freely usable resource for the industry. Over time, it is the intent of The Sequoia Project that this document is periodically updated to capture and advance cross-organizational patient matching innovations.
Intermountain Health Care, a CCC member, is a sophisticated healthcare provider and payer, and has invested heavily in healthcare information technology. So when they started exchanging data with other organizations, they expected a reasonably high degree of success. They surprisingly only achieved a 10% success rate in accurately matching patient records across organizational boundaries. Through algorithmic performance and refinement, and data quality analysis, Intermountain and its partners gained significant initial improvement from 10%. Human workflow and data entry issues were next inspected, with an expected improvement to 90%, but actually only resulted in an improvement to 62%.
The remaining 38% error rate was analyzed in detail and found to break down into algorithmic issues, authorization/consent issues, network and IT issues, and other errors such as inconsistent encoding. These improvements resulted in a match rate of 85%. A number of best practices were then identified and implemented resulting in successful patient match rates of over 95%. These practices include systematically working with partners, addressing patient consent issues, proactively improving “fragile” identities, and addressing human workflow issues. An analysis of common patient matching traits is presented, along with a list of rules developed during the study. These rules are further analyzed in terms of completeness and uniqueness. The result was significantly improved match rates for Intermountain and its partners, and patient matching improvement strategies that can be replicated across the industry.
At the conclusion of the case study a number of lesson’s learned and best practices are shared. There are also suggestions to improve cross-organization patient matching success to greater than 99%, which include the use of a supplemental identifier, involving the patient in the identity management process, and more. We also provide a self-diagnostic tool, in the form of a questionnaire, to jump start a self-assessment of your own patient matching strategies.
We believe that more precise definitions of the maturity model will give organizations the ability to adopt more advanced patient identity management in a methodical manner. The levels currently being contemplated in our proposed maturity model, now open to public comment, include:
Assessments are based on the International Organization for Standardization (ISO) framework, which includes people, process, and technology, with the added dimension of governance. Current traits associated with the various levels include patient identity management validation plans, community collaboration, use of standards, quality metrics, knowledge sharing, partner onboarding maturity, and more. Each of these traits is defined and mapped to appropriate maturity levels.
This proposed framework illustrates a very low-level, and concrete, list of cross-organizational patient matching practices. The target audience for this, the last section in this paper, is senior technical staff responsible for implementing clinical document care summary exchange. A number of specific rules are presented, such as the prohibition of using exact character-by-character matching, the corresponding responsibilities on both partners to an exchange of patient data, and similar practices. Other principles include not relying on any specific identifier (such as a Social Security number), not making any assumptions about the life cycle of a patient identifier, using normalized traits, and more.
Copyright © 2025 The Sequoia Project. All rights reserved.